Engaging Big Data
Jan 2016 - Dec 2020
Big Data-driven agent-based transport simulations
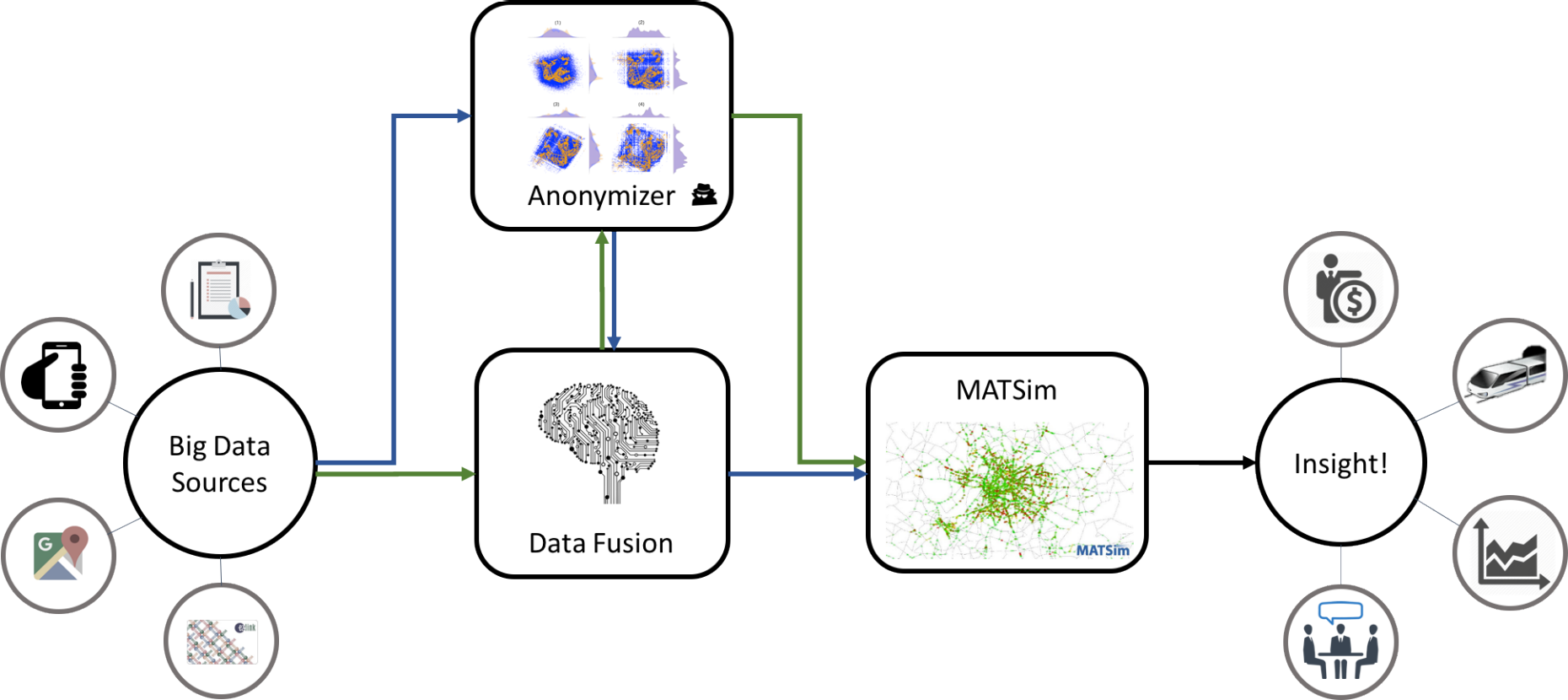
Traditionally, disaggregate demand modelling has been useful in explaining travel behaviour and forecasting travel demand. However, the biggest challenge in disaggregate demand modelling has perhaps been the reliance on a few observations of (usually) reported human behaviour. Recorded in travel diary surveys, these observations are later generalised to the population at large. The reliability of the model predictions are compromised when uncertainties or errors occur in this process of generalisation, and when these inaccuracies further interact and compound. Moreover, surveys are very expensive to conduct, take a long time to encode and release, and are only performed periodically, further compounding problems of generalisation with the added dimension of time.
With the advent of big data, from sources like transit smart cards and cellular phones, comes the potential to revolutionise disaggregate travel demand modelling by overcoming many of these problems. These data sources are congruent with the granularity and mode of analysis of the disaggregate modelling approach, providing direct observation of individual travel behaviour between activity locations on a daily basis.
In the Engaging Big Data research project, we build on the research that started in the first phase of FCL, where we developed a simulation of transit entirely driven by smart card data. In this phase, our aim is to extend our scope to include a wide array of big data sources, including mobile phone data. We fuse these with travel survey data to derive a complete and constantly updated travel demand description based on the actual behaviour of commuters.
We develop novel machine learning methods that can associate activity purpose and sociodemographic information with commuter trajectories. In order to ensure privacy, we investigate generative techniques that can produce endless streams of commuter trajectories that are, while not linked to specific individuals, but are realistic and representative of the population, and which will allow the system to be deployed in a ‘machine-eyes-only’ mode. The project also aims to address implementation challenges, including distributed simulation, and automatic calibration of simulation parameters.